Defining Big Data and How We Use it
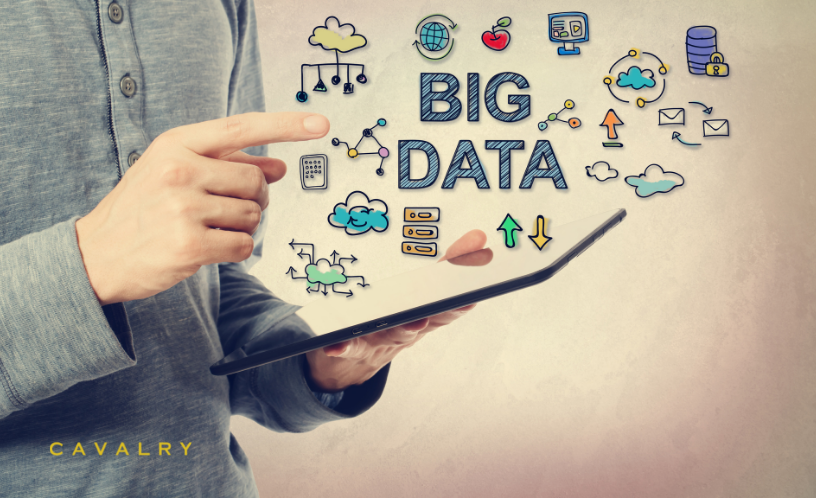
Hello everyone, it’s Brian, now Dr. Pendleton, again. Of course, for Ross and the Cavalry team it will always just be Brian. I’m back to write a bit more about data (and big data) and how it affects a model’s ability to help make predictions. If you need a quick recap on models, just give my previous post on machine learning a read.
In that post I mentioned the term big data, but didn’t really define it – so, let’s do that now. Much like the term AI (artificial intelligence), it doesn’t have an exact definition. However, most people in the industry accept Doug Laney’s definition, which uses the three V’s:
Volume, or the amount of data we have access to.
Velocity, or the growth in the amount of data being collected.
Variety, or the different types of data that we collect such as property values, videos, databases, and spreadsheets.
Today, many people include another V, veracity, in the definition as well. The veracity of the data is its truthfulness or quality. It’s what allows Netflix to recommend shows to you, Google to serve you ads when you search a term, and Tesla to enable its Autopilot feature. All those services get better as the AI is fed and trained on more information about you and others that are comparable to you.
Veracity is the reason that not all AI applications require millions or billions of records to train models on. Teaching a model to predict commercial real estate taxes in Washington, D.C., using data from Seattle won’t work. The data must be relevant to the jurisdiction the property is in. The same goes for features that could go into training the models being used. Stay tuned for a post on feature selection for models, but for now think of features as a list of everything that you have data captured for that can help train a model to make a better prediction.
Since a given jurisdiction only has a limited number of records for a property, you might be thinking it wouldn’t be enough to train a model. However, don’t think in terms of amount of data; instead think about the quality of that data. True, if there were only 200 or even 500 data points, trying to create a predictive model probably wouldn’t be the best use of resources. Thankfully, when it comes to real estate there’s decades worth of information (including many different kinds and sources) that impact property values and their subsequent taxes.
It’s all part of the secret sauce we call Taxonics. By combining the most relevant features, identified with the help of the decades of experience the Cavalry team has in real estate tax, we create the models that allow for the better, faster, and more affordable service we hang our hats on.
BACK TO ARTICLES